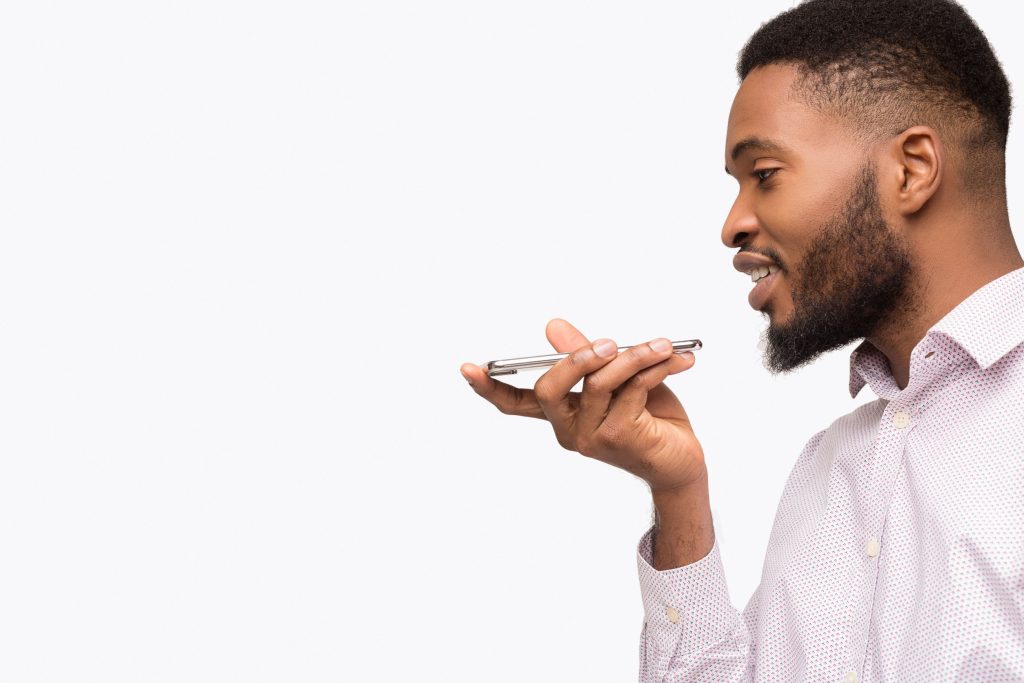
Accepted
AfriNames: Most ASR models “butcher” African Names
- 24th Interspeech Conference, Interspeech '23
Abstract
Useful conversational agents must accurately capture named entities to minimize error for downstream tasks, for example, asking a voice assistant to play a track from a certain artist, initiating navigation to a specific location, or documenting a diagnosis result for a specific patient.
However, where named entities such as ”Ukachukwu” (Igbo), ”Lakicia” (Swahili), or ”Ingabire” (Rwandan) are spoken, automatic speech recognition (ASR) models’ performance degrades significantly, propagating errors to downstream systems. We model this problem as a distribution shift and demonstrate that such model bias can be mitigated through multilingual pre-training, intelligent data augmentation strategies to increase the representation of African-named entities, and fine-tuning multilingual ASR models on multi- ple African accents. The resulting fine-tuned models show an 86.4% relative improvement compared with the baseline on samples with African-named entities.