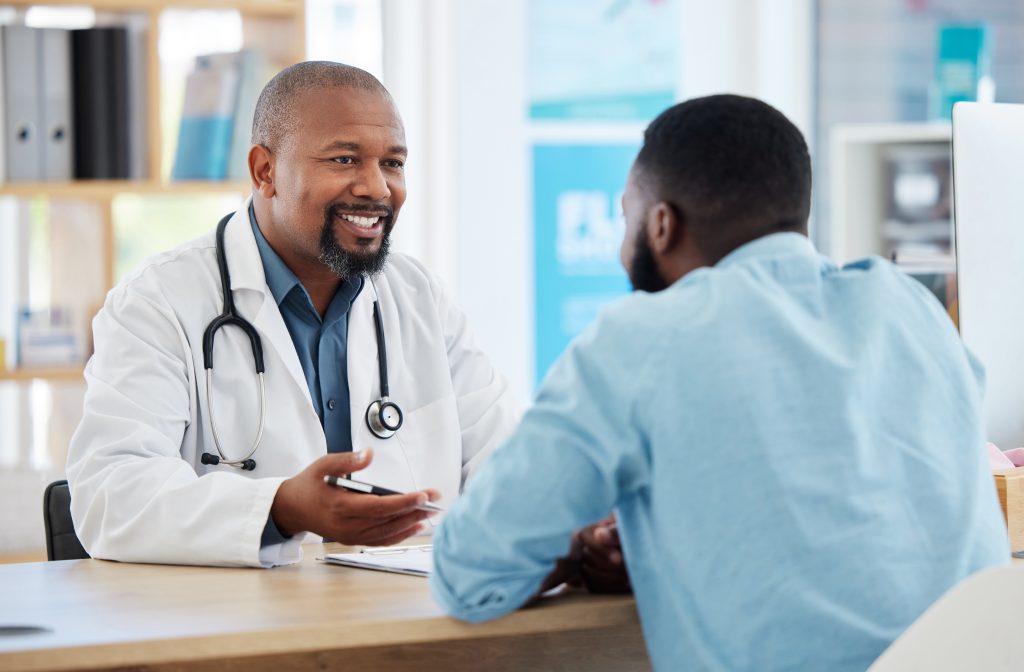
Under review
Afrispeech-Dialog: A Benchmark Dataset for Spontaneous English Conversations in Healthcare and Beyond
- NAACL 2025
Abstract
Speech technologies are transforming interactions across various sectors, from healthcare to call centers and robots, yet their performance on African-accented conversations remains underexplored. We introduce Afrispeech-Dialog, a benchmark dataset of 50 simulated medical and non-medical African-accented English conversations, designed to evaluate automatic speech recognition (ASR) and related technologies. We assess state-of-the-art (SOTA) speaker diarization and ASR systems on long-form, accented speech, comparing their performance with native accents and discover a 10\%+ performance degradation. Additionally, we explore medical conversation summarization capabilities of large language models (LLMs) to demonstrate the impact of ASR errors on downstream medical summaries, providing insights into the challenges and opportunities for speech technologies in the Global South. Our work highlights the need for more inclusive datasets to advance conversational AI in low-resource settings.