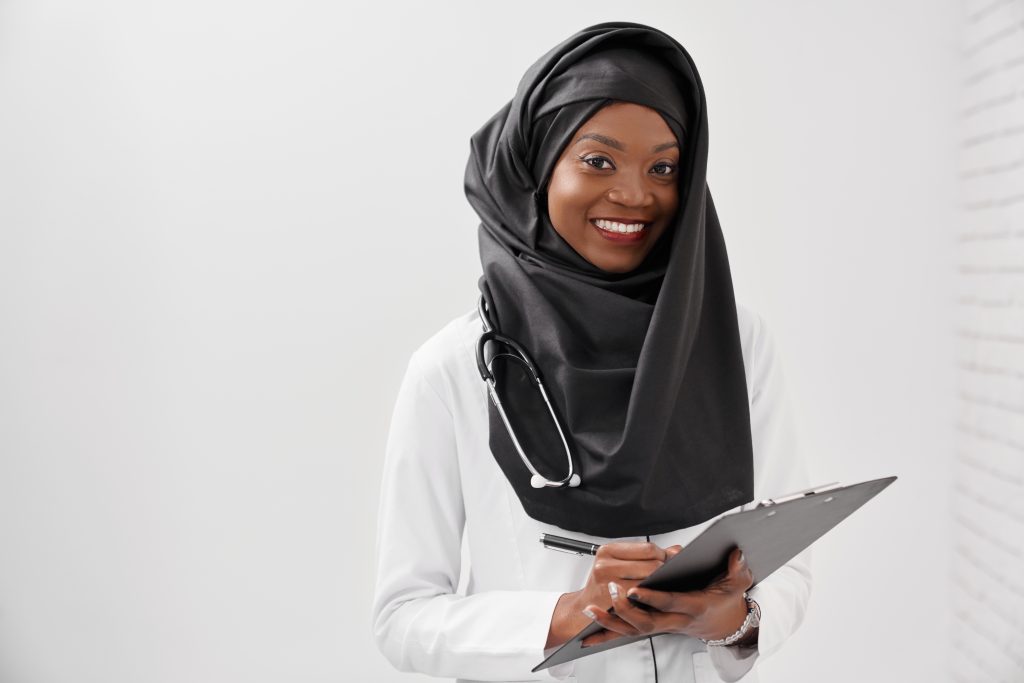
Under Review
Building Cost-Efficient, Robust and Linguistically Diverse Automatic Speech Recognition Systems in Pan-African Clinical Context
- 61st Annual Meeting of the Association for Computational Linguistics (ACL'23)
Abstract
Building robust ASR systems in this domain requires large amounts of annotated data for a wide variety of linguistically and morphologically rich accents, which are expensive to create. Our study aims to address this problem by reducing the annotation cost through informative data selection using active learning. We show that incorporating epistemic uncertainty into our active learning training loops achieves state-of-the-art results while reducing the amount of labeled data and annotation costs by 40% ($130,000+). Our approach also improves out-of-distribution generalization for very low-resource accents, demonstrating the viability of active learning for building generalizable ASR models in the context of accented African clinical ASR, where training datasets are predominantly scarce.