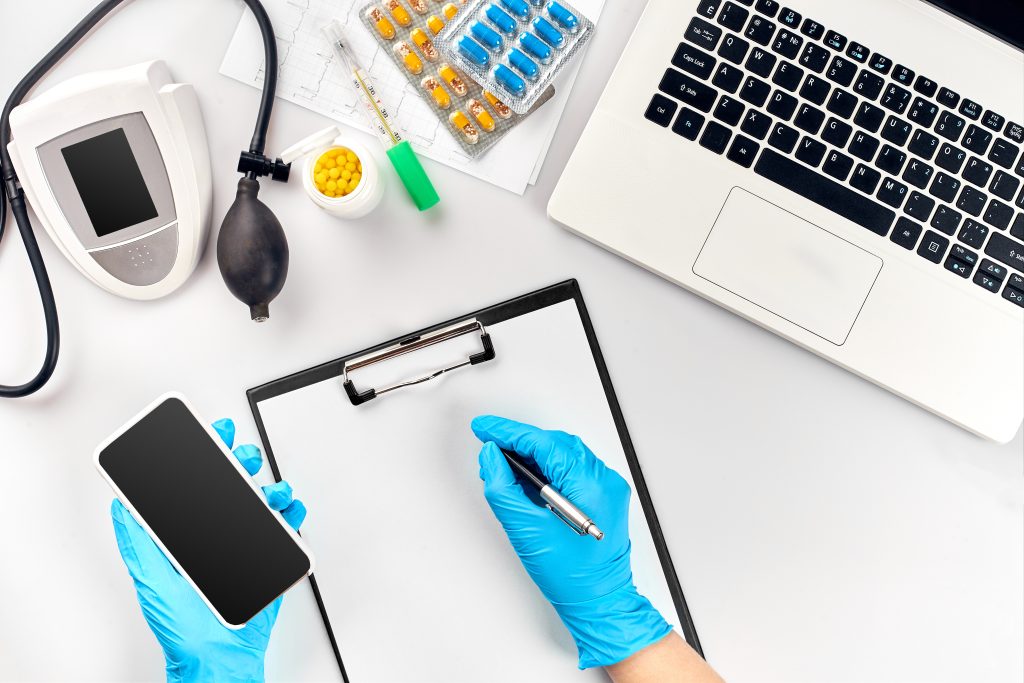
Accepted
Performant Medical Named Entity Recognition from Accented Speech
- 25th Interspeech Conference, Interspeech 2024
Abstract
Recent strides in automatic speech recognition (ASR) have accelerated their application in the medical domain where their performance on accented medical named-entities (NE) such as drug names, diagnoses, and lab results, is largely unknown. We rigorously evaluate multiple ASR models on a clinical English dataset of 120 African accents. Our analysis reveals that despite some models achieving low overall Word Error Rates (WER), errors in clinical entities are higher, potentially posing substantial risks to patient safety. To empirically demonstrate this, we extract clinical entities from transcripts, align ASR predictions with these entities, and compute Medical NE Recall, Medical WER, and Character Error Rate, novel metrics specifically developed to assess medical NE Recognition performance in speech. We demonstrate that finetuning on accented clinical speech improves Medical-WER by a wide margin (36% absolute), improving their practical applicability in healthcare environments