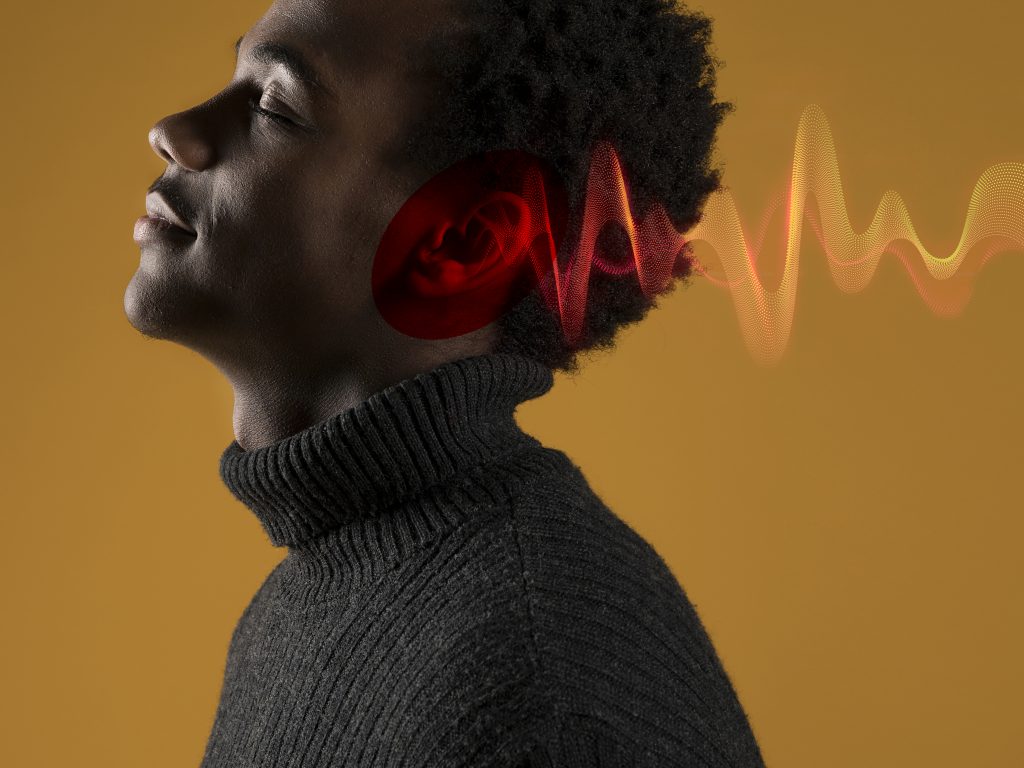
Under Review
AccentFold: A Linguistic Journey through African Accents for Zero-Shot ASR Adaptation to Target Accents
- Empirical Methods in Natural Language Processing '23
Abstract
Our work, in contrast, explores the under-explored area of African accent linguistics. We propose AccentFold, a method that utilizes learned accent embeddings to explore linguistic regularities between accents. Our exploratory analysis of AccentFold provides insights into the spatial relationships between accents and reveals that accent embeddings group together based on geographic and language family similarities, capturing phonological, and morphological regularities based on language families. Furthermore, we reveal two interesting relationships in some African accents that have been uncharacterized by the Ethnologue. Through empirical evaluation, we demonstrate the effectiveness of AccentFold by showing that selecting accent subsets for training based on AccentFold information outperforms random selection for zero shot ASR on target accents. With an average WER improvement of 3.5%, AccentFold presents a promising approach for improving ASR performance on accented speech, particularly in the context of African accents, where data scarcity and budget constraints pose significant challenges. Our findings emphasize the potential of leveraging linguistic relationships to improve zero-shot ASR adaptation to target accents.